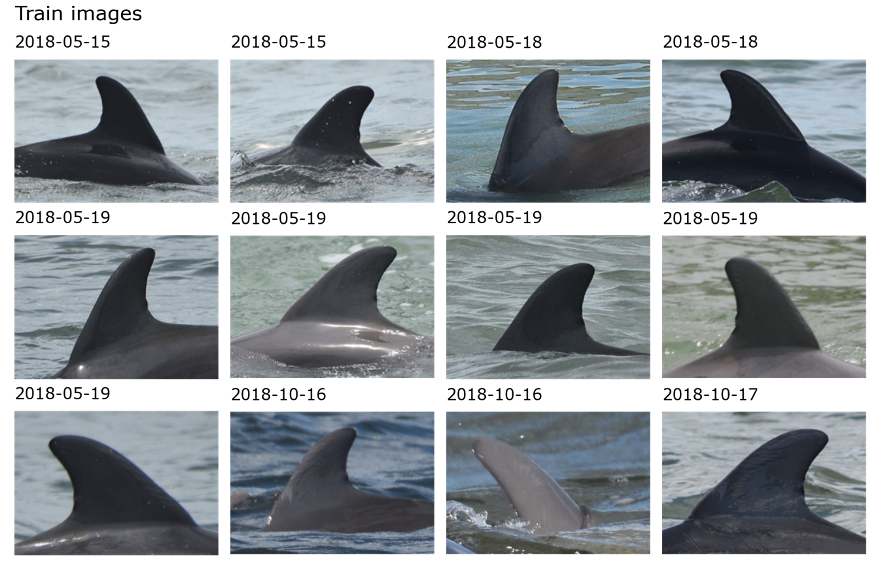
We are pleased to share a new publication in the journal Methods in Ecology and Evolution. The publication is the product of a massive collaboration, with researchers from around the globe sharing their valuable image data—representing six continents and 25 species—to advance cetacean research and conservation.
The manuscript is entitled: A deep learning approach to photo–identification demonstrates high performance on two dozen cetacean species
Authors: Philip T. Patton, Ted Cheeseman, Kenshin Abe, Taiki Yamaguchi, Walter Reade, Ken Southerland, Addison Howard, Erin M. Oleson, Jason B. Allen, Erin Ashe, Aline Athayde, Robin W. Baird, Charla Basran, Elsa Cabrera, John Calambokidis, Júlio Cardoso, Emma L. Carroll, Amina Cesario, Barbara J. Cheney, Enrico Corsi, Jens Currie, John W. Durban, Erin A. Falcone, Holly Fearnbach, Kiirsten Flynn, Trish Franklin, Wally Franklin, Bárbara Galletti Vernazzani, Tilen Genov, Marie Hill, David R. Johnston, Erin L. Keene, Sabre D. Mahaffy, Tamara L. McGuire, Liah McPherson, Catherine Meyer, Robert Michaud, Anastasia Miliou, Dara N. Orbach, Heidi C. Pearson, Marianne H. Rasmussen, William J. Rayment, Caroline Rinaldi, Renato Rinaldi, Salvatore Siciliano, Stephanie Stack, Beatriz Tintore, Leigh G. Torres, Jared R. Towers, Cameron Trotter, Reny Tyson Moore, Caroline R. Weir, Rebecca Wellard, Randall Wells, Kymberly M. Yano, Jochen R. Zaeschmar, and Lars Bejder
Scientists can learn many things about the ecology, social behavior, and demographics of wild populations—all critical study areas for conservation—by simply observing, recognizing and documenting the same individual animals over time.
One method for doing so involves capturing animals in the wild, applying some identifiable mark to them like a tag, then returning them to the wild. This method, of course, is invasive and can be ineffective without tagging many animals, a problem for large animals like whales and dolphins.
A popular alternative involves taking photographs of animals in the wild then trying to identify them, using their natural markings, on your computer screen in the lab. With this technique, scientists can learn about these animals and inform their conservation while minimally bothering them.

Recognizing the same animal in two different images, however, often requires time, money, and expertise, all resources that conservation organizations may lack. As a result, researchers have tried to automate this process with artificial intelligence and machine learning. These tools often need tens of thousands of images to be effective and, as such, have typically been developed for more common species that might be less of a conservation concern.
In our recent paper, we described and evaluated a new machine learning tool that proved to be effective on many different species of whale and dolphin, even rarer species. This tool works by applying a state-of-the-art method in human facial recognition to the backs and fins of whales and dolphins. Interestingly, it does so in such a way that the model is able to use what it learns about recognizing individuals of one species and apply it to other species. This is, perhaps, analogous to using what you learned about French in school to learning Spanish later in life.
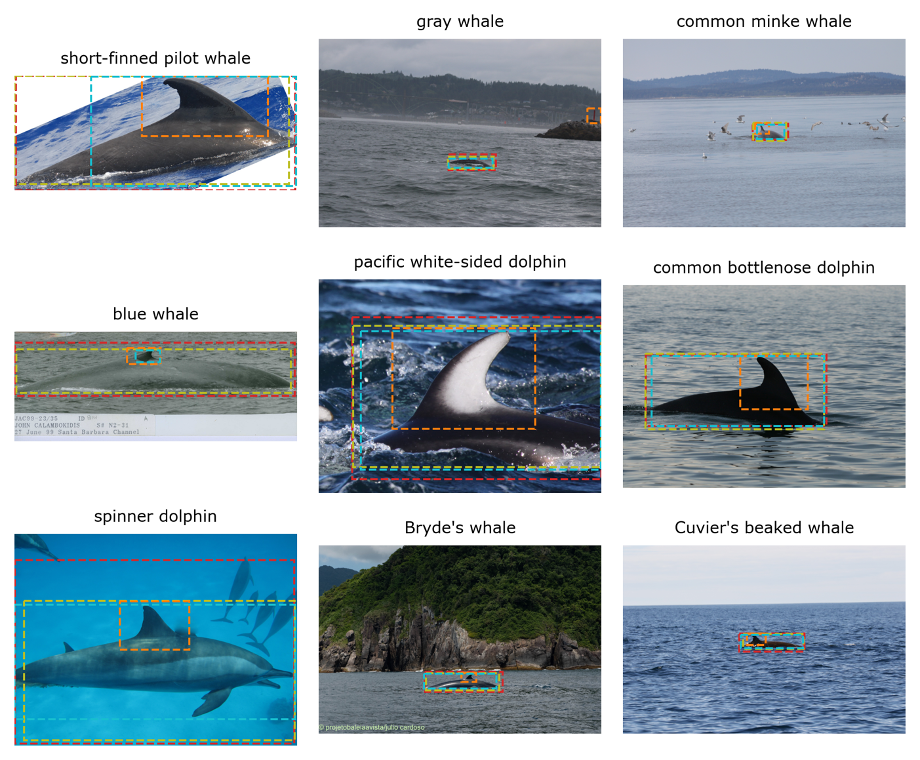
The model performed well overall, but the performance depended on the species and the image. One group of species—whales and dolphins with prominent fins on their backs—performed particularly well, even though some of the species individually had very few images. The model wasn’t magic though; it struggled to identify animals in pictures that were blurry, taken from far away, and/or contained beaching animals.
Using the lessons we learned about species-level and image-level performance, we outlined recommendations for future users of the algorithm. We hope that this guidance will help researchers understand how this algorithm might work, or not work, for their study area and species.
In many ways, this paper represents a win for collaborative and open science. The algorithm is freely available as code as this GitHub repository. A version of the algorithm has also been incorporated into Happywhale.com, a web interface for identifying animals and collaboratively sharing data. Additionally, the development of the model was only possible because dozens of research groups around the world agreed to contribute their valuable data to this project. These data are now freely available at Kaggle, the host of the competition whose winners developed the algorithm in the paper.
Patton, P. T., Cheeseman, T., Abe, K., Yamaguchi, T., Reade, W., Southerland, K., Howard, A., Oleson, E. M., Allen, J. B., Ashe, E., Athayde, A., Baird, R. W., Basran, C., Cabrera, E., Calambokidis, J., Cardoso, J., Carroll, E. L., Cesario, A., Cheney, B. J. … Bejder, L. (2023). A deep learning approach to photo–identification demonstrates high performance on two dozen cetacean species. Methods in Ecology and Evolution, 00, 1– 15. https://doi.org/10.1111/2041-210X.14167
Comments